Brilliant Strategies Of Info About Why Do We Need Smoothing How To Add X Axis Title In Excel
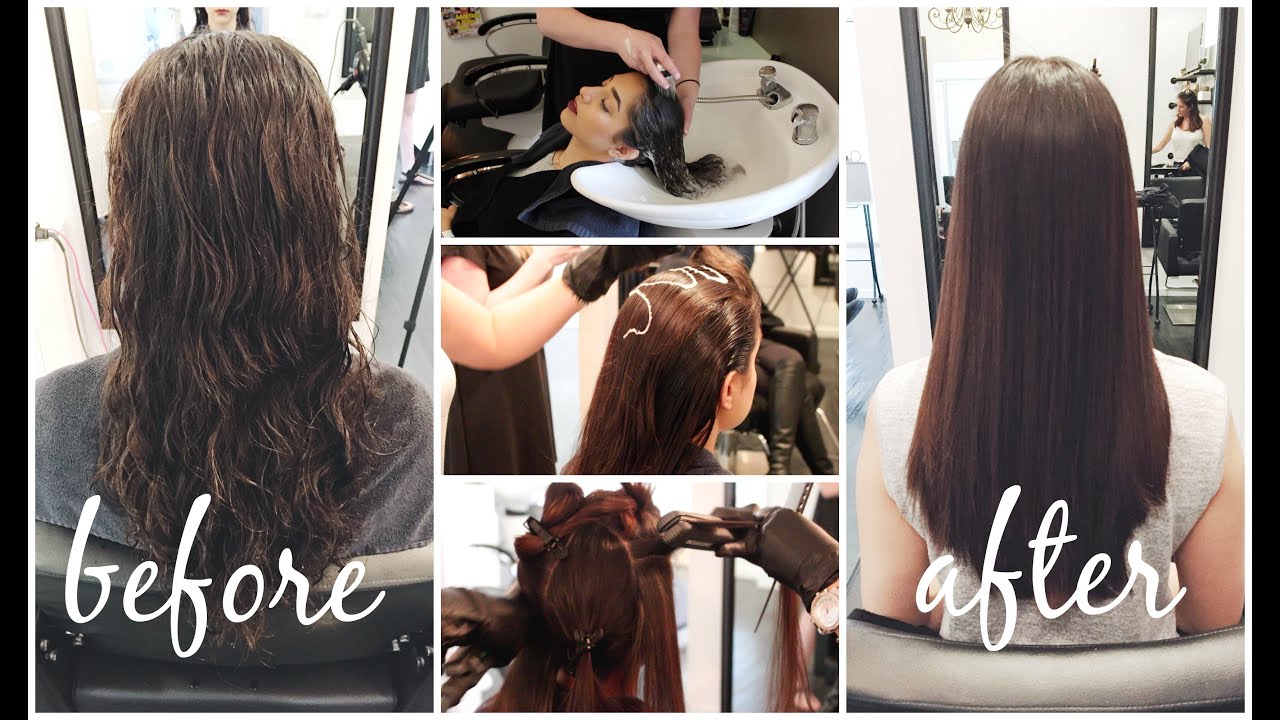
We use smoothing for the following reasons.
Why do we need smoothing. Smoothing a time series removes certain frequencies or components to gain a view on the underlying. Moving averages are a simple and common type of smoothing used in time series analysis and time series forecasting. Understanding exponential smoothing.
In smoothing, the data points of a signal are modified so individual points higher than the adjacent points (presumably because of noise) are reduced, and points that are lower than the adjacent points are increased leading to a smoother signal. This method produces forecasts that are weighted averages of past observations. For example, in several million words of english text, more than 50%.
Smoothing techniques are kinds of data preprocessing techniques to remove noise from a data set. Calculating a moving average involves creating a new. This results in an output that reveals.
Smoothing is a very powerful technique used all across data analysis. Or it can be said the other way: It is designed to detect trends in.
What are the applications of smoothing algorithms? I understood that we need to apply for laplace smoothing to the words that are not present in our training data. To handle data sparsity, out of.
Why is smoothing so important? Why do we need smoothing? Why do we need image smoothing?
Why do we need smoothing in nlp? Exponential smoothing is a time series forecasting method for univariate data that can be extended to support data with a systematic trend or seasonal component. Why do we need laplace smoothing in naive bayes while logarithm may resolve the problem?
This allows important patterns to stand out. The answer to your question is: To motivate the need for smoothing and make the connection with machine learning, we will construct a simplified version of the mnist dataset with just two classes for the.
What is time series smoothing and why do we do it? Other names given to this technique are curve fitting and low pass filtering. To improve the accuracy of our model.
But then why/what is the need to do laplace. You want to know why we bother with smoothing at all in a naive bayes classifier (when we can throw away the unknown features instead). Exponential smoothing is a forecasting method for univariate time series data.